- By quade
- 27 February 2024
LPU Chip Used By Groq AI Offers Record Performance
One of the newer AI models to enter the market was Groq, Elon Musk, the CEO of X. This program received widespread attention for its supposed advanced features and its ability to swear. But perhaps there is another thing that might set it apart.
The Language Processing Uni or LPU has made waves for its unparalleled performance compared to other AI tools of comparable size. There is hope that this system can provide new levels of efficiency in the AI market.
How Groq Was Tested
During a benchmark held by ArtificialAnalysis.ai, Groq was pitted against eight other AI platforms. All these models have their key performance indicators tested, including latency vs. throughput and total response time.
In many of these categories, Groq proved to be an excellent system, achieving a response time of 241 tokens per second. The developers also claim that under the right circumstances, it may reach up to 300 tokens per second.
This is far above other competing platforms, estimated to be double the speed of other platforms. The sheer speed of Groq may open possibilities for other models as it proves that these high speeds are not only possible but achievable.
Specs for the Groq LPU
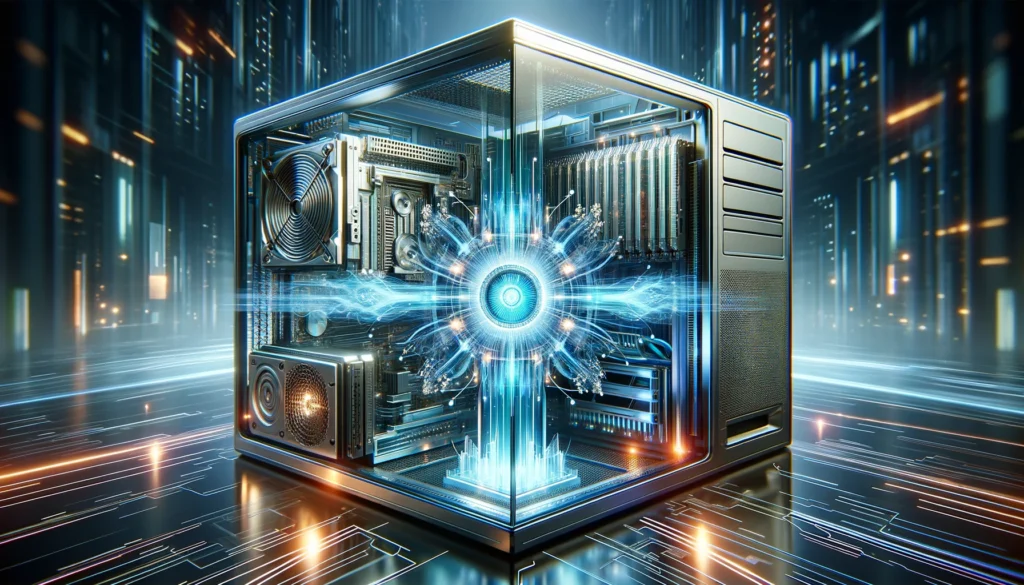
The main piece of hardware that makes these speeds possible is the GroqCard Accelerator. That piece is estimated at around $20,000 and is available for regular consumers. The LPU uses 750 TOPs (INT8) and 188 TFLOPs (FP16 @900 MHz) which boosts its performance. It is accompanied by 230 MB SRAM per chip and up to 80 TB/s on-die memory bandwidth.
Together, chips allow Groq to outperform the standard CPU and GPU setups, specifically in LLM tasks by significantly reducing the computation time necessary and the risk of external memory bottlenecks. This allows you to create text sequences faster than ever before.
The Groq LPU was often compared to NVIDIA’s flagship A100 GPU in terms of cost. Where the Groq LPU surpasses NVIDIA is with its speed and efficiency. A100 can enjoy a boosted performance, that can surpass the FP16 used by Groq in precision.
Evolution of Computer Components for AI
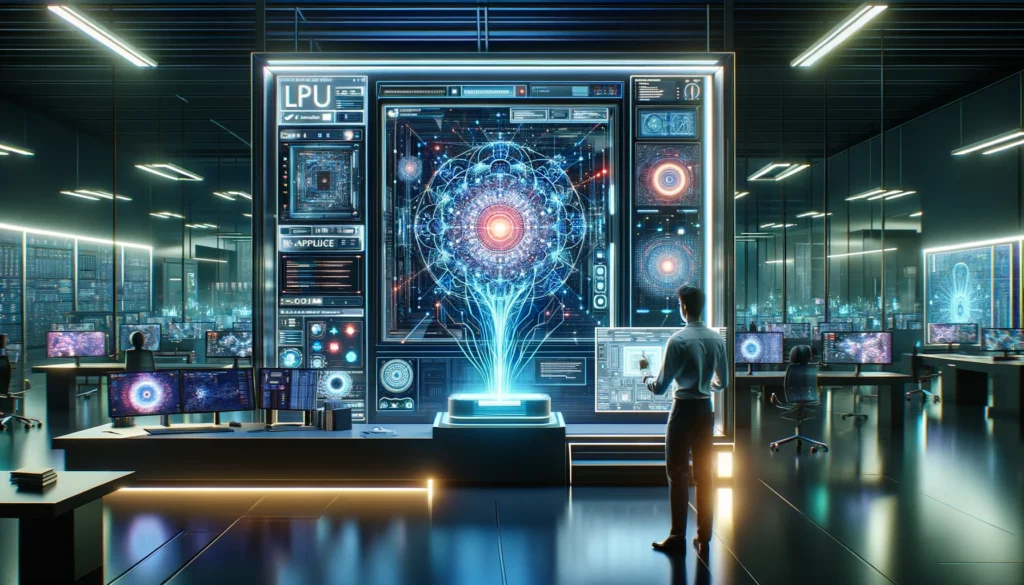
Groq’s new LPU could represent a milestone in computing hardware. The components used in PCs, such as CPU, GPU, HDD, and RAM have not seen any changes since GPUs were introduced. These GPUs were used to offload and accelerate the rendering of 3D graphics, making them critical in the function of large language models and other activities like gaming and computing.
As AI technology began to develop, the services GPUs offered also expanded there. These components became essential in AI usage because they can perform multiple operations simultaneously. However, while they were useful, there have not been any meaningful changes in the setup of these systems, and have remained as general-purpose computing.
This is where Groq LPU has changed things by addressing the need for these large language models. LPUs are designed with the tasks of AI in mind being custom-made to fit those needs. This allows a more streamlined approach for hardware when it comes to handling AI functions.
The biggest difference comes in the LPU’s increased compute density and bandwidth. Because of that, the systems can process texts faster.
The LPU now offers a specialized approach that focuses on optimizing the processing capabilities of LLMs. These can provide advantages in privacy, security, and efficiency compared to the API cloud services that other models like ChatGPPT use.
These differences come from CPUs and GPUs relying on external RAM for memory. This memory functions by being integrated into the chip directly which allows for high rates of data transfer.
In contrast, Groq LPU uses on-die memory of about 80 TB in bandwidth. This will allow them to handle huge data requirements faster and more efficiently. Experts believe that the creation of hardware specific to the LLM needs will go a long way in helping the AI market. There is hope that this LPU could lead the way for more innovative AI parts that can handle specific parts of the AI workload, taking the strain off overworked GPUs.
With the development of superior technology and components, we will take full advantage of these tools and add them to our hardware.