- By quade
- 27 March 2024
MIT Scientists Discover Method to Create AI Images Faster
Image generation has represented the biggest advances in the ever-evolving landscape of artificial intelligence (AI). New AI models can generate startlingly realistic images, representing a huge advancement from crude and nonsensical AI images.
However, generating these images has been a significant bottleneck as the process takes time. While faster than hand-drawn or digitally made images, it can still take the AI a long time to create an image, based on its complexity.
That was until the groundbreaking discovery by scientists at the Massachusetts Institute of Technology (MIT) which promises to speed up the creation of AI images, potentially reshaping the landscape of digital art, virtual environments, and beyond. This is a major opportunity for businesses to receive digital assets faster and more efficiently. This breakthrough comes when the demand for rapid image generation is greater than ever.
Condensing The Steps to Image Generation
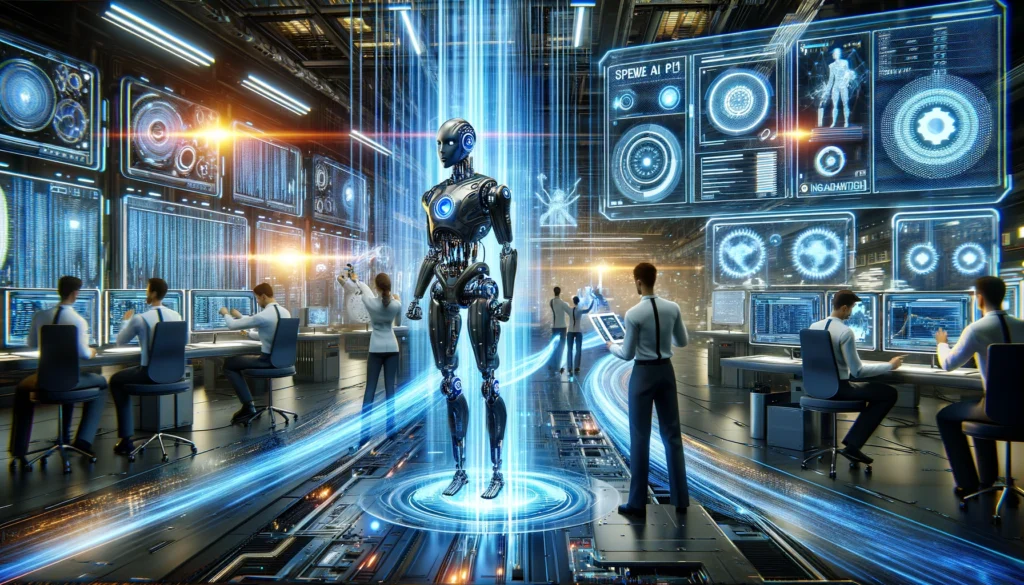
Recent research reveals that advancements in AI have allowed models to create images 30 times faster by using a process called “distribution matching distillation” (DMD). This condenses the normal 100-stage process into a single step.
DMD was developed at MIT and enables AI models to copy the uses of established image generators, such as DALL·E 3, Midjourney, and Stable Diffusion. By copying their framework, other AI models become more compact and efficient, generating images faster without sacrificing the image quality.
This was revealed in a breakthrough study published on Dec. 5, 2023, on the preprint server arXiv. This shows the promising potential of DMD in advancing AI-powered image generation.
One of the co-authors had some words about the success of his new process.
“Our work is a novel method that accelerates current diffusion models such as Stable Diffusion and DALLE-3 by 30 times. This advancement not only significantly reduces computational time but also retains, if not surpasses, the quality of the generated visual content.”
– Tianwei Yin, Co-Author
How Does DMD Work
Diffusion models use a multi-stage process to generate images. They are trained on data sets, including images descriptive text captions, and other metadata. These will allow the AI to understand what is in the image. When a prompt is placed, the AI scans prompts and matches it with the descriptions in its dataset.
According to AI scientist Jay Alammar’s blog post, these models operate by initially encoding a random image with a layer of random noise. This makes deciphering the image impossible for the AI but is part of a step called “forward diffusion,” which is pivotal in the training regimen.
The image will go through as many as 100 steps to remove the noise. This process is called “reverse diffusion,” which clears up the image and allows the AI to understand what is happening.
Their innovative framework streamlines the process by condensing the “reverse diffusion” steps into a single step.
DMD has two main steps to reduce the requirements to produce a viable image. The initial component, termed “regression loss,” categorizes images according to likeness during the training phase, to improve AI learning. The next step is “distribution matching loss,” which ensures that objects are depicted accurately to real-world counterparts. Together these reduce the number of steps required while maintaining quality.
Results of DMD
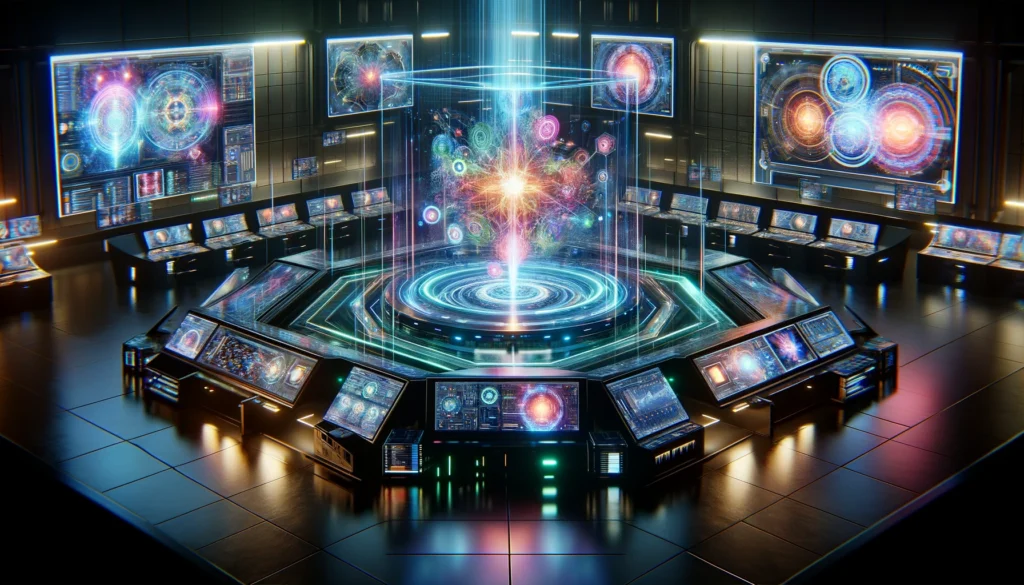
The test results of DMD are promising as their model decreased the image-generation time from around 2,590 milliseconds (equivalent to 2.59 seconds) utilizing Stable Diffusion v1.5 to 90 milliseconds — representing a speed increase of 30x.
The results have been exciting for the testing team as it opens many new possibilities for what can be done using image generation technology.
“Decreasing the number of iterations has been the Holy Grail in diffusion models since their inception. We are very excited to finally enable single-step image generation, which will dramatically reduce compute costs and accelerate the process.”
– Fredo Durand, Co-Author
The innovative method significantly decreases the resources needed for image generation, requiring only a single step rather than the “hundred steps of iterative refinement”. This streamlined approach holds particular promise for industries where rapid and efficient image generation is a major part of their success.
That is why the implications of this success go to MIT and affect business as well. This accelerated image generation can translate into increased productivity and cost-effectiveness, enabling quicker turnaround times for marketing materials, product designs, and alternative designs.
These benefits extend to the programming world as it means less computational overhead, allowing them to out their resources in other parts of their process.
Creating assets and images is an important part of our programming service as we want to create the best-looking website for our clients. Our geniusOS team is now studying the potential benefits of the DMD process and how we can implement it. If you want to learn more about what our programming services have to offer, you can contact us here.